ALCOA is and mnemonic device1Wikipedia: A mnemonic device, or memory device, is any learning technique that aids information retention or retrieval in the human memory for the elements of data quality, particularly with respect to its use as evidence of regulatory compliance. The acronym was first used by Stan W. Woollen of the FDA’s Office of Enforcement in the 1990s2He tells the story of how it came to be in this SRCSQA newsletter article.. ALCOA stands for Attributable, Legible, Contemporaneous, Original, Accurate.
The European Medicines Agency (EMA) added another four items to round out the concept: Complete, Consistent, Enduring and Available3I believe it was in the 2010 “Reflection paper on expectations for electronic source data and data transcribed to electronic data collection tools in clinical trials”, though I have no evidence that this was the first use of ALCOACCEA. This highlights data management requirements that were somewhat implicit in the original five. We commonly refer to this extended concept as ALCOA+.
Introduction
In this article we’re going to take a deep dive into ALCOA+, starting with what the regulations and guidance have to say on the topic. Then we’re going to explore each of the nine aspects of ALCOA+ in detail with a few illustrative (if not always realistic) examples to help us on our way. Though much of the context I’ll give comes from a GLP perspective, it will be applicable to quality systems in general.
The Motivation
Whether you’re working with non-clinical safety studies, clinical trials, pharmaceutical production, forensics, air traffic control software or medical devices, your product’s quality is directly linked to public safety. Consequently we as a society have decided that we require evidence to support that quality.
In a physical product such as pharmaceuticals or medical devices, the measure of product quality might be in meeting a specification, or in statistical terms such as the number of defects per batch. You might measure software quality in terms of test coverage or defects per line of code. For GLP studies and GCP trials, the product is the final report and we measure its quality in terms of the data supporting the report’s conclusions.
Quality of Evidence and Reconstruction
We need to have enough evidence to be able to reconstruct4Notice I’m not using ‘reproduce’ here. Although in GLP we like to talk about reproducibility (a hold-over from our analytical backgrounds, perhaps?), we very rarely have the need to reproduce a GLP study but very often reconstruct them. the production of the batch, the conduct of the study, or the commit history of the software.
Who did what and when? What procedures, materials and tools did they use? Were the materials and tools fit for their purpose? While each discipline might differ in what they measure as product quality, how you measure the quality of evidence is the same in each case, and this is what ALCOA+ describes. In fact, ALCOA+ is a very succinct framing of the core principles of Good Documentation Practices.
But First… I Want My Mnemonic Back
ALCOA. Does it sound familiar? That’s probably because it also happens to be the name of the world’s largest producer of aluminum. So it’s memorable, and has an obvious spelling from its pronunciation. That makes it a good mnemonic. A mnemorable mnemonic, if you would.
Useful as the extensions to ALCOA may be, ALCOACCEA just doesn’t seem nearly as memorable as the original. And though I might remember ‘ALCOA-plus’, I’m never sure what ‘plus’ is meant to stand for. I need an ear-worm, something to make ALCOACCEA stick for good. So let’s fix that right now, with a standard pronunciation and a bit of a rhythm, you know, to make it roll off your tongue:
I agree, that really wasn’t worth the time it took to create. It’s a good thing I’m in lock-down. On the plus side, you’re likely never going to forget that, right? Good. I’m going stick to calling it ALCOA+ for this article, but I want you to say it out loud, with rhythm, every time.
Where Is It Written…?
First things first. There aren’t any direct references to the acronym ALCOA or ALCOA+ in, for example the FDA or EPA GLPs, or in the OECD Principles of Good Laboratory Practice. I don’t believe it features in any of the U.S., EU or Canadian GMPs or GCPs either. For the longest time it just sort of appeared5Apparently it first ‘sort of appeared’ because Woollen ran out of room on a slide: “… I do remember the consternation of at least one member of the audience, who in trying to later decipher the “government jargon” in my slide, asked what ALCOA stood for.” here and there in conference presentations and training decks.
You could say that knowing what ALCOA stood for was an indicator of who you’d been hanging around.
…In Regulatory Requirements
While the acronym “ALCOA” doesn’t itself appear in the regulations, each of the underlying concepts are well represented in the regulations. If we take a look at the paragraph in the FDA GLPs on study conduct, 130(e), for example, it basically lists requirements for Attributable, Legible, Contemporaneous, Original, Complete and Enduring records (ALCOCE), as the following colour-coded reading demonstrates:
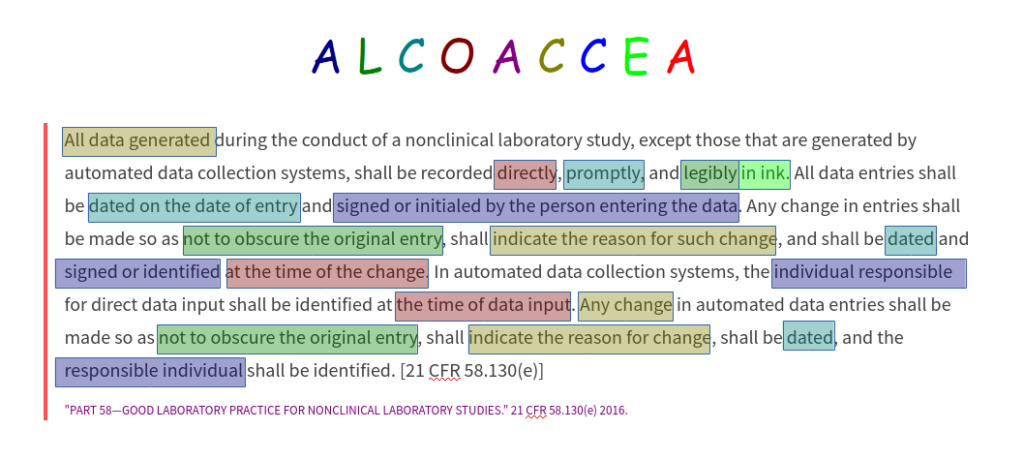
Furthermore, you can easily find clear requirements for Accurate, Consistent and Available records in the paragraphs on equipment (§61, §63) and archiving (§190). Note that the corresponding paragraphs 130(e) from the FIFRA and TSCA GLPs are almost identical.
The study conduct section of the OECD Principals of GLP is very similar:
8.3.3) All data generated during the conduct of the study should be recorded directly, promptly, accurately, and legibly by the individual entering the data. These entries should be signed or initialled and dated.
8.3.4) Any change in the raw data should be made so as not to obscure the previous entry, should indicate the reason for change and should be dated and signed or initialled by the individual making the change.
8.3.5) Data generated as a direct computer input should be identified at the time of data input by the individual(s) responsible for direct data entries. Computerized system design should always provide for the retention of full audit trails to show all changes to the data without obscuring the original data. It should be possible to associate all changes to data with the persons having made those changes, for example, by use of timed and dated (electronic) signatures. Reason for changes should be given.
“OECD Principles on Good Laboratory Practice.” OECD-GLP-1-98-17.II.8.3 1998.
Although they tend to be spread out a little more, you’ll find similar requirements in the various GMP and GCP regulations as well. I’ll leave it as an exercise for you, the dear reader to colour code your own copy of the regs.
Since it’s mentioned in the the OECD quote, I’ll take a brief moment to point out that the terms Raw Data (GLP) and Source Data (GCP) are variously defined by the regulations and guidance documents. For our purposes they are equivalent and mean the original record of the original observation. We will cover this a little more later on.
…In Guidance Documents
Over the past decade ALCOA began showing up in written guidance from various authorities. For example, ALCOA is mentioned in both the FDA’s 2013 Guidance for Industry: Electronic Source Data In Clinical Investigations, and the FDA’s 2018 Data Integrity and Compliance With Drug CGMP: Questions and Answers Guidance For Industry. The 2017 ISPE GAMP Guide: Records and Data Integrity has a section on ALCOA and ALCOA+. The mnemonic is also prominent in the MHRA’s 2018 GXP Data Integrity Guidance and Definitions.
Data integrity is certainly a hot topic these days. This is a little surprising to me, since I always thought that data integrity was the point of the GLPs from the beginning, right? Perhaps we got lost somewhere along the line and need to be reminded again. Looking at the FDA’s definition of Data Integrity from its 2018 cGMP guidance:
Data integrity refers to the completeness, consistency, and accuracy of data. Complete, consistent, and accurate data should be attributable, legible, contemporaneously recorded, original or a true copy, and accurate (ALCOA).
Data Integrity and Compliance With Drug CGMP: Questions and Answers Guidance For Industry. FDA (2018)
The MHRA pulls in the final four:
Data governance measures should also ensure that data is complete, consistent, enduring and available throughout the lifecycle […]
GXP Data Integrity Guidance and Definitions. MHRA (2018)
…In Findings
And yes, not only has data integrity been a hot topic in new guidance documents, it has also been a hot topic in inspection findings, at least in the U.S. Get this: 79% of warning letters issued by the FDA to the drug industry in 2016 cite data integrity deficiencies6“An Analysis Of FDA FY2018 Drug GMP Warning Letters” Unger, B. Pharmaceutical Online, Feb 2019.. Though the numbers were better for 2018, they were still above 50%. So this is definitely something we need to improve upon, as an industry.
This brings me to an important point. Remember that the tenets of data quality and integrity are enshrined in the regulations. ALCOA+ and its relatives are just tools. Whether the document or methodology you’re following calls it ALCOA, ALCOA+, ALCOA-C or ALCOACCEA, the data integrity requirements for the evidence of product quality (and the expectations of your monitoring authority) remain the same7The 2018 MHRA guidance (see ¶3.10) states this explicitly.
The Fundamental Tenets of Data Quality and Integrity
Let’s summarize what we have so far. ALCOACCEA stands for Attributable, Legible, Contemporaneous, Original, Accurate, Complete, Consistent, Enduring and Available. These are the core tenets by which the records of evidence of compliance with regulations are measured. These tenets translate directly to the notions of Data Quality and Data Integrity that are written into the regulations.
Now, measured implies evaluating something that already exists, which makes sense when you look at it from the perspective of an inspection agency or auditor. For our purposes we want to look at how we bias our systems to generate compliant records in the first place, by incorporating Good Documentation Practices.
ALCOACCEA by Example
Let’s delve a little deeper into those nine tenets. Here’s an overview of ALCOACCEA by example:
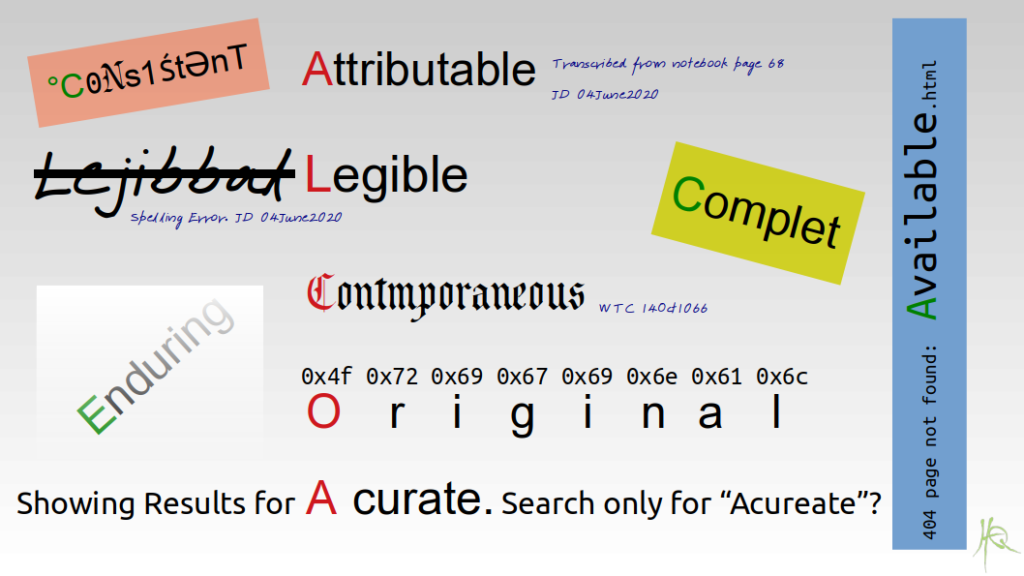
A – Attributable
In order to weigh the veracity of a record, we need to be able to attribute the record to its source8Here I use the word “source” as in “the source of that smell”. We’ll get to the defined term “Source Data” later on – but if we were to continue the analogy, the source data would refer to the undiluted, original smell.. If the source was appropriate, that lends weight to the data. This means the record must indicate: who recorded the data point; when it was recorded; and what the record represents.
Who and When
The who and when are pretty straight forward. For paper records the regulations dictate dated signatures or initials in wet ink:

Easy, right? You’ve probably had this drilled into you since first year chemistry, when they had you number, sign and date each page of your notebook. Note that there’s no mention of colour in the regulations, so I’ll leave it to you to argue over black, blue or chartreuse.
In electronic records it’s usually up to the system to support attribution, for example by electronic signature and an audit trail. Hybrid systems can be a little more tricky, since you need to consider the temporal and format disconnect between the record itself, the signature, and the audit trail.
Wait… What?
It might surprise some of you a little that I included what as part of attribution. Supporting data should be covered elsewhere, right, for example in the section on ‘Complete’, or ‘Accurate’? True, however I want to highlight that sometimes a record requires additional context at the time of recording in order to give meaning to the data. Annotating the attribution, for example by adding a note beside your dated initials, or adding a statement of intent to an electronic signature, might be the only option to record that context.

In general, we want to minimize the need for users to decide what context is necessary, and leave annotations to the truly unanticipated. In most cases we can build context into whatever recording system is in use. A well designed form and SOP can provide cues to ensure important details are not omitted. For example: we can write in prompts for units, ids and other contextual data right into our forms and SOPs.
Regardless, it is good to remember that in the absence of context, details must be provided by the user, and this need is going to come up eventually. The system should provide for this need even if it’s just by providing space or procedures for comments and additional details.
It’s On Your Permanent Record
An attributable record should allow someone to link the signature back to the person. For example each study or facility should have on file samples of the signatures and initials of study staff and any contractors. This allows inspectors and auditors to verify that the source of the record was, for example, appropriately qualified.
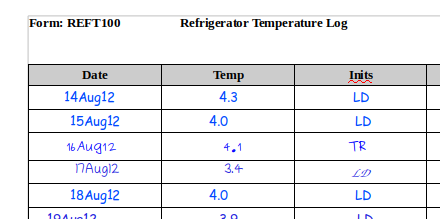
Perhaps a 3-letter standard for initials is called for?
The rules around using initials for identification should allow for people to be uniquely identified. Likewise, changes in a person’s signature or initials (for example, due to legal or preferential name changes) should be recorded in the system, with a clear indication of when those changes came into effect.
Premeditated Attribution
While the act of attribution does seem straight forward, what you don’t want is for staff to have to guess, on the fly, which data points need dated signatures. Even well trained talent, at the end of a long shift, will eventually convince themselves that a whole table of observations taken over several hours only needs to be signed and dated once.
Build attribution into your forms. Include prompts for context, and provide for unexpected details, for example through annotation procedures or comment fields. Evaluate software, including that for electronic signatures, for how it supports all aspects of attribution. Ensure hybrid systems are well described in your SOPs, and that any disconnect between the record and its signature are handled appropriately.
L – Legibility
Records need to be legible, and to remain so through their useful life. Paper records can sometimes present a legibility challenge, especially when there are handwritten comments. A well designed form will definitely improve legibility. Electronic records are often encoded in a format that’s not human readable, and then would need software to make them legible, for humans at least.
The requirements for legibility, however, go beyond taming your doctor-scrawl and being able to view gifs of cats in party hats. For example, the original recording, its attributions and metadata, and any subsequent changes to these (and their attributes and metadata and changes… and so on) must remain legible throughout the life-cycle of the record.
The Thin Blue Line
When making any corrections to raw data, do not obscure the original entry. For handwritten data, place a single line through the previous entry, and ensure each correction is properly attributed, including, for example an initial, date and a clear reason for the change. As discussed above, a standard method for annotating marginalia can improve the overall clarity of handwritten records and forms.

Electronic systems should always retain the original recording as an immutable record and provide a complete audit trail of any changes. Consequently the legibility of electronic records often depends on the data format and the software support for that format. How a system handles the legibility of and changes to raw data is critical, and should be considered during the early design evaluation and validation phases of any new system. User requirements, specifications and testing should include tests for raw/source data immutability, data change control and audit trails.
C – Contemporaneous
Contemporaneous means ‘timely’. Our memory is volatile: the image of an observation decays as time goes on. As a result the more promptly an observation is recorded, the better the quality of that record. Therefore, data should be recorded as they are observed, and the record should include a time9I use time here to include both time of day and the date. Which needs to be recorded for a particular application will depend on what you’re recording. entry matching the time of the observation. The more support for contemporaneous recordings the system provides, the better.
This analogy of memory being volatile extends to computerized systems as well: for example, the signals from a detector are electrical impulses whose result must be evaluated and recorded before the signal is gone. We don’t usually have to worry about such details unless designing an instrument. However it’s worth remembering that even in computerized systems, the observation and the creation of the record are separate events.
Filling it in by Hand
Avoid temporarily jotting down results on a scrap of paper, post-it, napkin or the back of your hand and then transcribing it to the ‘official’ form. If you do, remember that the form is not Original nor Contemporaneous, and you really should be signing, dating and archiving the back of your hand.
When a data point is measured, immediately record it in the available field. Ensure that all information required by the form or SOP is also recorded. For individual data, write each data point at the time that it is read, rather than reading multiple points and then writing them down as a group. For batch data, take the time to verify each point as it is recorded.
A great way to improve legibility and thereby reduce the need for draft versions of forms is to constrain the options for certain responses. For example, if there are only two options for an answer (Yes/No or Pass/Fail, etc.), then don’t make the person write out the whole word. Use design elements such as check-boxes or letter abbreviations to make it as easy as possible to fill out the form correctly, the first time. On the other hand, be careful when using check-boxes that you don’t end up with ambiguous states: for example, does an unticked checkbox mean ‘No’, or does it mean the user missed that question?
Speaking of Your Backhand…
Do not backdate records. If something is a late entry, then state so and give a reason for why it is late. This may well be a deviation or non-compliance, however it’s better than fraud. You may need to justify the source of that back-filled data as well. This allows the Study Director, auditor or inspector to determine if it is a piece of data that was legitimately determinable after the fact, rather than relying on your memory or hearsay.
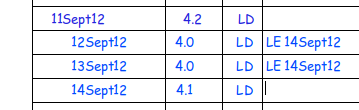
However, you need to make sure any back-filled data is valid!
How is LD sure that the temperature was 4.0 on both of the days that were missed?
Watch out for systems that pre-fill dates for you beside the places to sign, they will get you in trouble. As a general rule if you’re signing in ink, then you should probably ink the date as well. If you’re using an electronic signature, that signature should have a contemporaneous timestamp associated with it.
Employ a Helping Hand
Ensure all raw data is recorded in real-time, as displayed, and according to an approved SOP or protocol. Write procedures to encourage this. If a procedure cannot be completed properly by one person while also recording the results, then require an additional person to be present to do the recording. Dosing, for example, might be done in pairs with one person keeping time and records. This also allows you to easily build in verification steps, for example to double check IDs and volumes.
Finally, synchronize clocks so that timestamps flow in a logical order. If one is easily accessible, you might want to define a central source for synchronizing against. Account for format considerations such as timezones and daylight savings, especially when combining data from several disparate sources.
O – Original
As we discussed above, data should be recorded as they are observed. We define the equivalent terms ‘raw data’ (GLPs) and ‘source data’ (GCPs) to mean the original recording of an original observation or activity. If contemporaneous to the observation, these original records are considered to be the the point of highest quality because they have been subjected to the least amount of manipulation. Manipulation potentially introduces errors, for example, in transcription, translation or interpretation.
Therefore, you must keep the original recorded form of the data, if possible. Verified copies may be used in place of the original, if for good reason (for example to create an Enduring record). However, this should follow an SOP for making such copies, including full documentation of the process.

Unless it’s absolutely obvious, define what the raw data is for each system or procedure. This will make it easier to reason about where, when and how the original data will be generated, ensuring its content and meaning are preserved. Identify transcribed data and date, initial, and document the original source.
The Little White Lies that Robots Tell Us
Defining the original raw data becomes especially important in the case of computerized instruments. There is often a big difference between the human readable version of the data (for example, what’s presented to you on the screen) and the actual raw data being recorded.
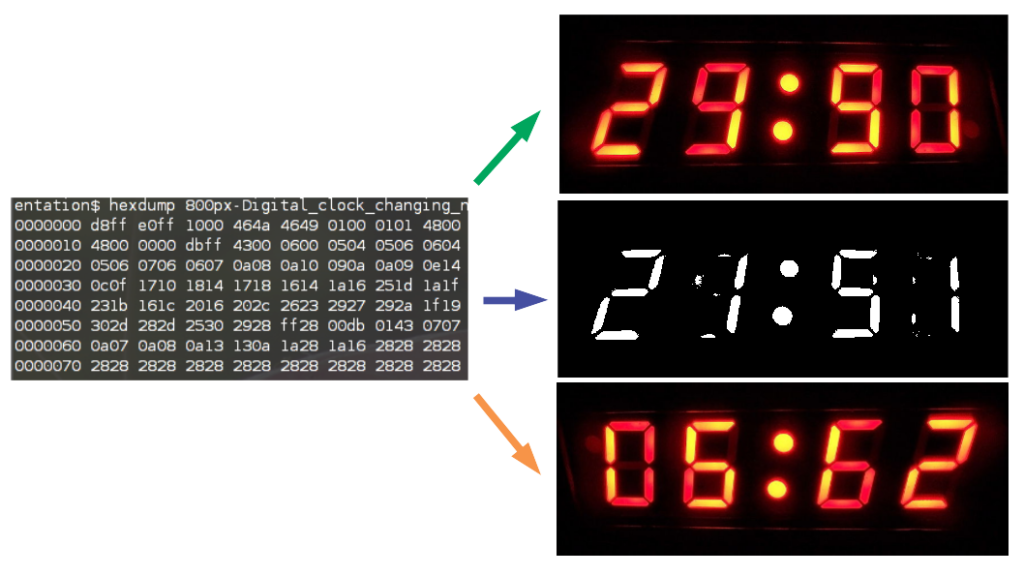
On the right are examples of ‘human readable’ representations of the same file, from top to bottom: (1) colour monitor (2) black and white monitor (3) upside-down monitor.
If we take a look at the image file of a digital readout, above, we can see that without changing the underlying raw data, we can get a completely different ‘human readable’ result. In this case, by changing the monitor (or settings in the image viewing software) we see a completely different apparent result: 29:90, 21:51, or 6:62, whatever that means. Which is the true representation? In fact, each of these are interpretations of the raw data and not the raw data itself. In this case the SOP for interpreting the results should specify the monitor or software configuration to use, and the raw data needs to be kept in case it ever needs to be reinterpreted.
Why Keep the Original?
Moving to an example that’s a little closer to reality, let’s say you are operating an HPLC to measure the concentration of a drug in a sample. In this hypothetical system the software might show you, on the screen, a table of areas vs. retention times, along with a chart of the integration. You review the data, give it a title, and press ‘Continue’. The system prints out what you see on the screen, and the table data is written to an Excel file that gets automatically uploaded to the network to be held in a Laboratory Information Management System (LIMS).
The actual raw data that was recorded by the instrument, however, might be in the form of voltages (representing what was actually measured by the detector) and integers (whole numbers representing the computer’s internal definition of time). These numbers are saved in a binary format to a file or database along with some metadata providing, for example, the start time of the integration and the sample ID, etc.
That table of data that was saved to the spreadsheet is what you, the operator, first see. It’s what you use for processing, analysis and reporting. Not only have you never even seen that original binary data, if you did it would be largely meaningless to you. So why should you keep it? Why not treat the human readable version as the raw data and archive that?
You Can’t Unbake a Cake
As we saw with the digital readout example, that first human view of the data might be after many processing steps that are dependent, for example, on software settings. If there was some kind of misconfiguration you could always reprocess the raw data. You can lose or mess up an Excel file and easily recreate it.
In many cases you can’t go the other way. This is because during data processing information might be aggregated, narrowed or translated in a way that loses information.
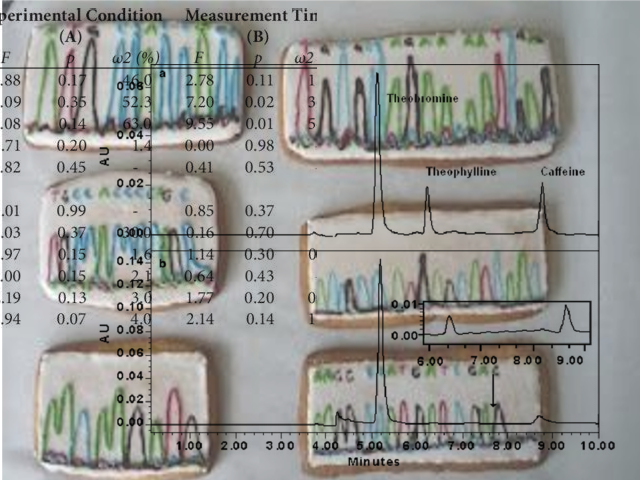
Processed data always needs to be traceable back to the original observation: the raw data. Make sure you’ve identified and retained (read: archived) the raw data and its metadata, and make sure it is Available for audits and inspections.
It’s Not Always Obvious
There may be circumstances where it’s not obvious which version of the data to treat as the original. Or the original is not practically accessible. For example, some temperature/humidity recorders only provide access to their internal data storage through some intermediate software. Maybe the software provides several options for exporting the data, none of which are obviously (nor likely to be) an exact copy of what’s stored on the device. In such a case it’s important to identify (and validate, as appropriate) which of those options you’re going to treat as the raw data. Put that in your SOP. Depending on the criticality of the data, you might want to consider a different device.
A – Accurate
Since we are talking about data integrity, it probably goes without saying that the record should be accurate. As we learned above, the original, contemporaneous record is the point of highest quality because it has been created before any errors or biases could be introduced. Said another way, we cannot add quality once we’ve made this original observation. It’s all downhill from here!
That’s why that original observation is so critical. Use a system that minimizes errors, ensuring that raw data are correctly presented. This means calibrated instruments, validated computerized systems, and quality control processes that are fit for the immediate purpose.
Promptly and directly record the actual observation. Don’t truncate data, or add decimal places. Data entry systems should validate form submissions, but not modify the user’s input. Any auto-fill or auto-correct features of the platform should be turned off.
Form Validation
Validating a form field basically means that the software checks that you’re not making any obvious mistakes or omissions. It warns you of those before the data is submitted. Think about the last time you filled out a signup form online. If you wrote something in the email address slot that didn’t have an ‘@’ in it, the form would highlight the field in red and display an error message. What you don’t want, is for the form to ‘correct’ the spelling of your email address to something else and submit that data instead.
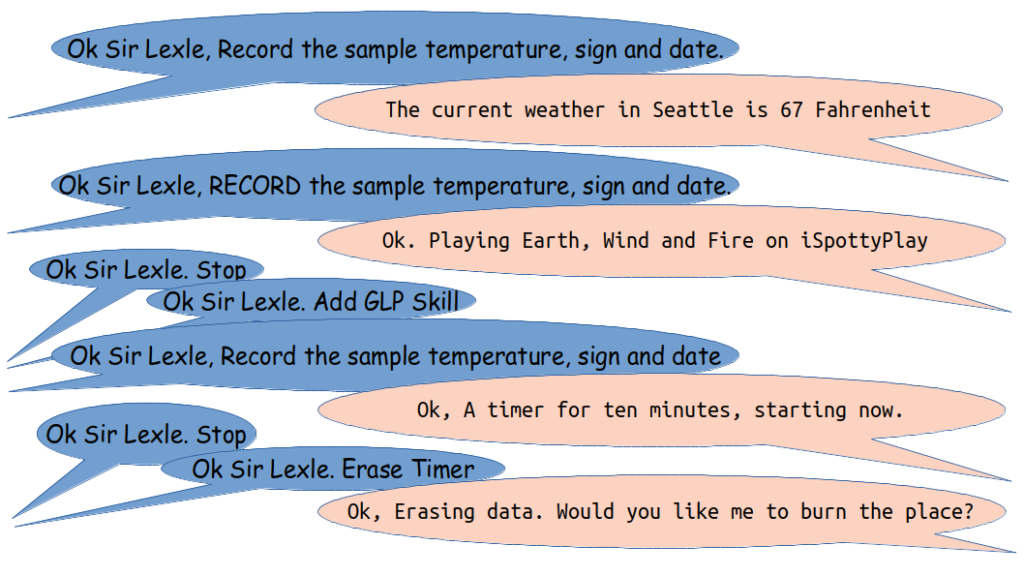
Web technologies and platforms have grown beyond being used just for browsing the internet. Because they work across devices and are quick to develop, they have become popular as front-ends to databases, whether the database is on the internet, network or on your local workstation. Data input and reporting front-ends in life sciences applications are no stranger to this – I’d bet that most of the software in your data workflow have already moved to a web based front end, at least for configuration and reporting.
Auto fill and auto correct are features that that are turned on by default in many modern web platforms. They can be a significant problem in badly designed forms that haven’t annotated their fields properly. Increasingly, issues related with ‘helpful’ features such as auto correct have the potential to creep into your data flow.
The Trouble with Tribbles
Copies of digital media may be created relatively easily and on a large scale. Without careful organization, multiple instances may lead to questions as to which is the correct, original record. Furthermore, it is very easy to propagate errors or otherwise unintentionally change files or their metadata while copying.
Be very careful of systems or procedures that have the potential to generate multiple, slightly different versions of a record. Where possible, use tested and documented systems for moving and copying original records. Similarly, use approved media for data storage and have some quality control over any data transfer across different systems to ensure that an exact copy is made.
Trust, but Verify
There are many methods for verifying copies after transfer. For example, for small human-readable files you could visually verify the contents along with its file size and/or metadata. For binary data or numerous files, you can use fingerprinting methodologies such as checksum/hashing routines to compare the copied files to the originals. Do not depend on file size and metadata on their own as a check of data integrity.
In any copy or transfer operation, ensure that the appropriate metadata is also copied, and if the metadata is a separate file object verify its integrity in turn.
Take special care when moving data to different media or to external systems. If the underlying file systems are different, the transfer may alter the data or its metadata in unexpected ways. Similarly check that transferring to the new format will not render the data unreadable without specialized equipment.
Always verify the interaction between the systems on either end of a transfer! If you’re interested in reading more on this, check out my write-up on the unfortunate and unintended outcome of moving a record though multiple automated systems that were each, individually, working exactly as designed.
C – Complete
The record needs to be complete. That means you must include all descriptions, metadata and associated information necessary to reconstruct the record. This is basically an extension of the what in Attributable, but also overlaps with many of the other sections.

In the example above we have a number, initials and a date. Great. Do we have ALCOA? The record by itself is, of course, meaningless because it lacks any context. Put yourself in the place of an auditor 5 years later. What does 41 represent? Who is TR and what role did they have in the study? Was this a record from 2012 or 2015 (or perhaps even 2008?).
Now, this might seem a little contrived, since I’ve intentionally removed the context. In most cases, the context would be obvious, right? Implicit in the record itself, for example in the form header or in the surrounding notes?
Removing the Guesswork
Let’s zoom out to view some of the surrounding context of our example:
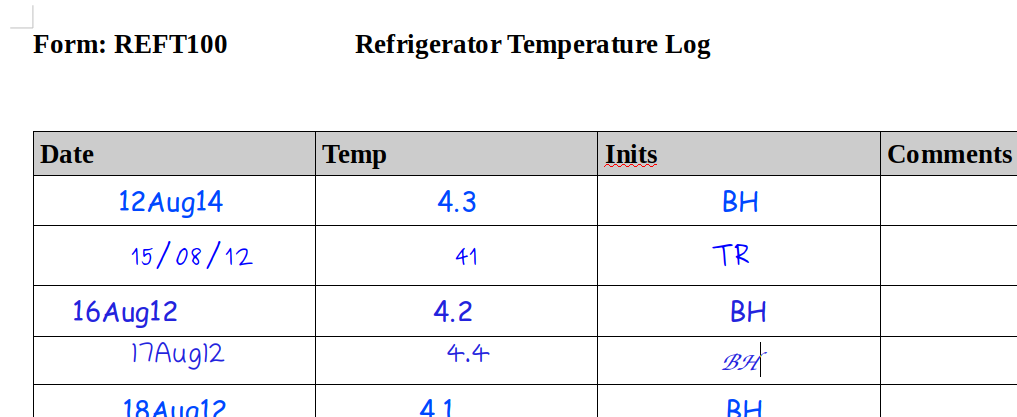
Ok, so now we see that this is daily temperature data for a refrigerator. There are no units specified – the form has an ID that will hopefully link back to the SOP associated with it, so perhaps we’ll get the units being recorded from that. There’s no equipment ID, again hopefully this is the only refrigerator in the laboratory. As you can see, there’s still a lot of guesswork necessary here to figure out the context of the data. The context also shows that there’s something very wrong with our initial record (the line filled in by TR). But what? Did they forget the decimal? Or did they record the temperature in Fahrenheit? And so on.
Metadata
We’ve mentioned metadata a number of times, but we haven’t really defined it yet. Metadata is information describing a piece of data – literally data about data. In regulated data it may include the initials, dates, times and other audit trail information; explanations and comments; setup information such as equipment settings, sequence files, etc. It may include attributes such as resolution, size or frequency. File permissions and date stamps. Anything that describes the data.
One of the things that concerns us about metadata is where it occurs. In a paper record system, the metadata may be written beside the data, or it may be in the marginalia. Or it may be in filing cabinet #3, room 25b in the basement of the corporate offices in Atlanta. File sizes and timestamps might be kept by the filesystem. Newer filesystems also include things like tags and image dimensions. Many instruments will store metadata about samples in a database or a separate file.
Even More Meta
A complete record also includes any linkages to other information that supports the quality and reconstruction of the data. We discussed above linking initials and signatures to a list of study staff on file. These in turn link back to the staff’s personnel file and training records, which in turn support that they are appropriately trained to collect the data in the first place. These linked data need to persist in the archives for the life of the record so they can continue to support the data if questions come up.
Similarly, records should be able to be linked back to the equipment used to produce them, including their validation state, maintenance and calibration records, and any configurations that were active during the recording. A temperature record that doesn’t indicate the thermometer used is for all intents and purposes meaningless. There is no way to link it back to whether the thermometer was within its calibration. A chromatograph with an ID that doesn’t link to a sample number would be similarly meaningless.
Close It Out
We’ve already talked of the advantage of designing forms that prompt for complete records. Another approach to ensure records are complete is to have a process for closing out records once they are completed. How you do this will depend on the type of record and your quality system.
Example strategies include ensuring blank spaces don’t have any default meaning to the completed record. Mandate the use of “NA” or lines through unused fields, and ensure these are initialled and dated. For electronic applications, form validation should enforce a complete record, and any default values be made explicit or preferably avoided. This means that if I don’t explicitly complete a field, the system refuses to move forward rather than assuming a value for the blank field.
Schedule a regular QC of forms and other records, for example at the end of the day, week or session. Include procedures for checking empty fields, and annotating any missed entries. Add signature requirements for QC and review of forms. Where appropriate, you could include an automated process for identifying gaps in electronic data.
C – Consistent
Records need to be consistent, both internally, within its immediate set, and with regards to the larger body of information. For example, records should be consistent with respect to order of generation, units, signing, procedures used, etc. Data should be gathered using a system that enforces the use of approved data acquisition and analysis methods, reporting templates, and laboratory workflows.
A Flawed Example
We’ve seen throughout our examples that having consistent policies on data formats improve the quality of the data. The other side of this is that inconsistency is a sign of deeper problems. Lets take another look at our very flawed refrigerator temperature log:
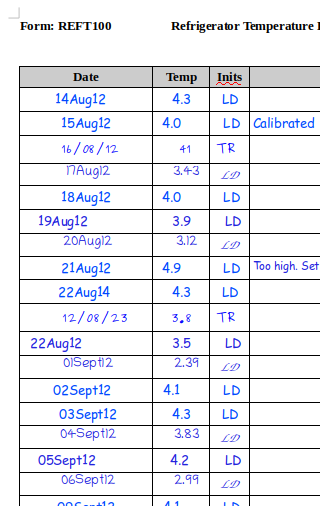
The date column shows not only inconsistencies in format, but also inconsistencies in date order. What happened at the end of August? Was this someone going on holiday, or was the refrigerator out of action? Either way this is at best an SOP deviation. If the refrigerator was storing test item for a GLP study that had to be kept at a certain temperature, it might be much worse.
The inconsistencies in the temperature column data are also very interesting. LD number two always records an extra decimal place. Is everyone else rounding off data in their head? Or is she using a different thermometer? Notice that her numbers are consistently lower than everyone else’s readings… and so on.
Unfortunately you’re not going to be able to do much to go back and fix this kind of inconsistency in your data. If caught early enough you can try to add explanations and complete any deviations necessary. If it’s caught too late all you can do is sit and watch the questions pile up from the auditors.
Standardize
Inconsistency, of course, is a sign of a lack of standardization. One of the first things that you should have in place to improve data consistency is an SOP on data formats. This would be the place where you specify your default date format and how other date formats in the body of records are to be resolved. This would also be the place where you would lay out your rules for interpolation and significant figures. For example, how do you handle reading ‘between the lines’ on an analogue bulb thermometer or graduated cylinder?
Equipment SOPs are also a good place to discuss data formats, especially where the equipment’s reporting is user configurable. Forms should either specify units or provide a clear area for recording the unit. The goal here is to have the data consistent across time, people, and even equipment models.
There’s a Time for Rounding
One caveat: your forms should never enforce units or the number of decimal points unless absolutely consistent with the instrument generating the data. Remember, your original data record should always be the original observation. Rounding is a manipulation of the data that can come later.
Where a system cannot be configured to match your chosen standard, ensure the format is documented. If necessary, establish a process (no matter how obvious) for translating that format to the standard at the time of processing or reporting. As always keep the original record.
E – Enduring
The record must not change or disappear over time. Now it should be obvious to all of us that pencils for recording raw data are right out. It wasn’t so long ago when certain equipment only spat out records on thermal paper, Polaroid film or other self-destructing media. Hopefully those days are behind us in the laboratory?
Yet we are not immune from having to consider the lifetime of records. Data formats change and the software to read them come and go. I still have a pile of zip disks somewhere, filled with AutoCAD (for DOS. Loved it.) projects on it that I was sure I’d revisit some day.
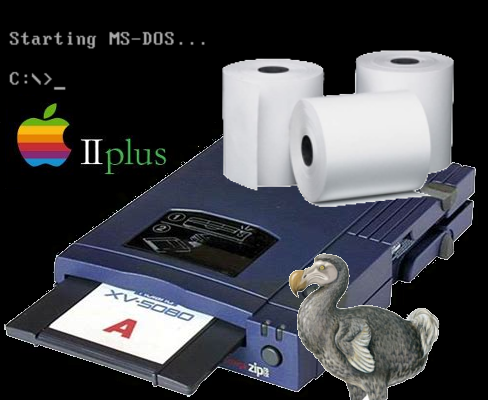
One of the big contributors to electronic records not ‘enduring’ is vendor lock-in. Proprietary formats and media often die with their profitability, and this means you need to plan how you’re going to access records in the future. For some data sources this may mean you need to take steps to ensure the data will survive archiving. Examples of such mitigations include making verified copies on other media, or storing software or hardware needed to access the data.
Above and Beyond the Call of Duty
Back a few years ago I attended a workshop on archiving electronic data. One of the people in my group told of her company’s two archived Apple IIe computers. Apple IIe came out in 1983, making it 37 years old at this writing. It was also less powerful than your toaster.
Apparently this company had years worth of very important data that could only be generated by what I’m guessing was some sort of custom built system. And the generated data could only be read by these venerable toasters. So, two archived units, one as a backup in case the other ever died. Every year these had been dutifully dusted off and started up, fingers crossed, tested, and then wrapped up and put away again for another year.
Evaluate every new computerized system for its raw data formats and compatibility. Make it a part of your requirements setting and initial evaluation of the system. What’s the raw data? What format is it in? What media is it going to be stored on? What’s needed to read that data? Are there any other compatibility issues?
A – Available
Last but not least, the record needs to be available when needed. ‘When needed’ might be for Quality Control (QC), processing and reporting, for a Quality Assurance (QA) audit, for inspectors, for sponsor review.
So what does it mean to be available? From the point of view of a regular inspection from the authorities, it probably means producing requested data in a legible format before the end of the inspection. However, there may be other considerations you need to make to ensure records are available within a reasonable timeframe.
There may need to be a mechanism to allow the Study Director and QA access to raw data at test sites. For binary data certain software might need to be available (and working) to view the data in human readable form. The use of off-site archiving facilities may require an agreement (for example, in the Service Level Agreement or SLA) as to what a reasonable access time to records might be.
Data retention and availability is a widely studied topic and way beyond the scope of this article. There are many guidance documents on archiving. When in doubt, enlist the expertise of an appropriately qualified archivist (a requirement for GLP facilities) or the IM/IT department. Any larger project that involves computerized systems handling regulated data should certainly do so early on in the process.
Conclusion: ALCOA+ by Design
We’ve seen that ALCOACCEA are the core tenets of data quality and data integrity, and that they come directly from the regulations. As we discussed each of these tenets, we built up strategies to build data integrity into our systems, allowing us to bias those systems to produce undeniable evidence of the quality of our products.
To summarize some of the strategies I discussed:
- Standardize attribution where possible, including how and where to record signatures, initials and dates, as well as annotating notes and changes:
- Evaluate software, including that for electronic signatures, for how it supports all aspects of attribution.
- Describe hybrid systems in SOPs, including how to link the record to its signature;
- Training programs should emphasize the proper way to use attribution and annotation features in software;
- Move away from free-form notebooks wherever possible and instead use structured forms to standardize recording of data. This also gives you many options for adding cues to ensure records are complete;
- Constrain response options where appropriate to make forms easier to fill out in a timely and accurate manner. Validate electronic form fields. Disallow empty fields;
- Design procedures to ensure observations are recorded as they happen. Incorporate recording of data into procedural steps. If this will take two people, then require two people;
- Provide methods to identify and link records and samples to their metadata, systems, people, instruments as well as any other supporting records, deviations or notes to file;
- A strong ID system also allows for linkages forward through data processing and on to reporting as appropriate;
- Conduct iterative form reviews and test runs during the design phase and at SOP review to ensure they encourage collecting the complete and accurate record;
- Perform an analysis of the raw data and metadata formats, audit trails and input controls of electronic systems as part of their validation. Measure these against ALCOA+.
Finally, if there’s anything I want you to take away with you today, it’s this: AAL-KOH-AAH-KAA-KEE-AAH. Say it with rhythm!